Abstract
Universities increasingly integrate online education into their pedagogical practices, utilizing digital tools like video conferencing and discussion boards to enhance learning and foster global collaboration. Research shows that technology influences social interactions and collaborative problem-solving, positively impacting student engagement, satisfaction, and academic performance. However, the debate on online education's benefits and drawbacks continues. Proponents highlight democratized access, flexible schedules, and cost reduction, while critics point to inadequate face-to-face interaction, technical challenges, and perceived lower credibility of online degrees. Despite these drawbacks, advancements in technology are mitigating some concerns. Additionally, mental health significantly affects academic success, but there is limited data on support systems for online students. This study examines the relationship between anxiety levels and academic performance among graduate students in an online counseling program. The findings revealed no significant relationship between anxiety levels and GPAs, challenging previous research and highlighting the complexity of factors affecting student performance in online education. This suggests that other variables, such as time management, support systems, or coping mechanisms, may play a more critical role. Further research is needed to explore these additional factors and develop comprehensive support strategies for online learners.
Keywords: online education, technology, learning communities, mental health, academic performance, anxiety, graduate students
Balancing Technology and Mental Health
The integration of online education into university curricula has rapidly expanded in recent years, driven by advancements in digital technology and the increasing demand for flexible learning options (Di Giovanni & Cronin-Gilmore, 2024; Beldarrain, 2006). Online education utilizes various digital tools, such as video conferencing, discussion boards, and multimedia presentations, to enhance learning experiences and promote global collaboration among students (Harvey‐Jones et al., 2022; Stephens, 2023). This shift towards digital pedagogy has sparked considerable debate regarding its effectiveness compared to traditional face-to-face instruction (Fedynich, 2013; LaPointe & Reisetter, 2008).
Proponents of online education argue that it democratizes access to education, allowing students from diverse geographical locations to participate in courses without the constraints of physical proximity (Fedynich, 2013; LaPointe & Reisetter, 2008). This flexibility is particularly beneficial for non-traditional students, such as working professionals and those with family responsibilities, as it accommodates their schedules and reduces the costs associated with commuting and on-campus living (Christensen & Eyring, 2011). Additionally, online education can leverage diverse technological tools to create interactive and engaging learning environments that support collaborative problem-solving and active participation (Zhao & Kuh, 2004).
However, critics of online education raise concerns about the lack of direct face-to-face interaction between students and instructors, which can hinder the development of personal connections and immediate feedback (Talebian et al., 2014). Technical challenges, such as unreliable internet connections and insufficient digital literacy among both students and educators, further complicate the online learning experience (Hamdan, 2014; Nkonge & Gueldenzoph, 2006). Moreover, the perceived lower credibility of online degrees compared to traditional ones continues to be a point of contention in academic and professional circles (Deming et al., 2015).
Beyond these pedagogical and logistical considerations, the mental health of students in online programs is a critical factor influencing academic success. Previous research has established a strong link between mental health issues, such as anxiety and depression, and academic performance in traditional educational settings (Barr, 2014; Keys et al., 2012; Kessler et al., 1995). However, there is limited empirical data on the specific mental health challenges faced by online students and the effectiveness of support systems available to them (LaPadula, 2003).
This study aims to explore the relationship between anxiety levels and academic performance among graduate students enrolled in an online counseling program. By examining this correlation, the research seeks to contribute to the ongoing discourse on the efficacy of online education and identify potential areas for improvement in supporting the mental health and academic success of online learners.
Literature Review
With technology rapidly changing and constantly upgrading, many universities have increasingly incorporated online education into their curricula (Di Giovanni & Cronin-Gilmore, 2024). Institutions are redeveloping their pedagogical practices to keep pace with evolving societal trends (Beldarrain, 2006; Lloyd et al., 2023). Traditional in-person lectures are now often supplemented with digital tools, such as video conferencing, PowerPoint presentations, online discussion boards, wikis, and blogs, enabling educators to reach a broader student demographic (Harvey-Jones et al., 2022; Stephens, 2023). This technological shift facilitates collaboration among students both within the classroom and globally (Hatahet et al., 2024).
Research indicates that technology significantly influences social interactions, which can directly affect the learning process (Bransford et al., 1990; Lave & Wagner, 1990). Collaborative problem-solving within learning communities is linked to improved student performance (Hu et al., 2012; Zhao & Kuh, 2004; Stassen, 2003; Pike, 1999). For instance, Zhao and Kuh (2004) examined the relationship between learning community participation and student engagement across 365 four-year institutions, finding a positive correlation between learning community involvement and overall satisfaction, engagement, and self-reported academic outcomes. Similarly, Stassen (2003) discovered that first-year students in cohort-based learning communities achieved better academic results.
A key finding in recent research is that students in online learning environments desire a sense of belonging (LaPointe & Reisetter, 2008; Shae & Bidjerano, 2010; Shae, 2006; Pez, 2010; Hatahet et al., 2024). Collaborative activities and student-led discussions are crucial for enhancing performance in online education. Moreover, educators' technical skills are essential for facilitating effective learning (Sanda, 2024). Shae and Bidjerano (2010) identified a strong correlation between the online learning community and students' self-efficacy, emphasizing the importance of teacher and social presence in achieving successful learning outcomes. Shae (2006) also noted that a stronger sense of connectedness within the online community leads to higher academic achievement, with increased instructor participation further enhancing students' connection to the community (Sanda, 2024).
Evaluating the Benefits and Drawbacks of Online Education
Both institutions and researchers have debated for decades about the advantages and disadvantages of online education (Fedynich, 2013; Pelz, 2010; LaPointe & Reisetter, 2008; Zhao, 2004; Stassen, 2003; Pike, 1999). The general theme amongst those in support of online education is that it allows students from several areas across the world access to the same education as a student located in close proximity to the institution (Fedynich, 2013; LaPointe & Reisetter, 2008; Zhao, 2004; Giokaris, 2024). However, on the opposite side, many researchers argue that online education lacks face-to-face time between instructor and learner (Fedynich, 2013; Stassen, 2003; Pike, 1999). The following sections will provide an in-depth analysis of studies weighing both the benefits and drawbacks of distance learning.
Benefits of Online Education
With online higher education becoming more prevalent at universities nationwide, there have been numerous advantages to universities, educators, and students. Many theorists have postulated that distance learning allows students to attend classes without being within the same vicinity of the institution (Fedynich, 2013; Beldarrain, 2006; Rezaei, 2024; Baron et al., 2023)
Technology allows for more remote locations to have similar access to education as those students in more populated areas (Beldarrain, 2006). Students can work at their own pace and form online learning communities with one another (Lapointe & Reisetter, 2008; Tao et al., 2006; Shea, 2006; Zhao, 2004; Stassen, 2003). Also, institutions reported that distance learning has been a way to offset the rising costs of higher education (Arkoful & Abaidoo, 2015; Fedynich, 2013).
In past years, location has been a significant barrier to equal access to education. With current technology, we can serve a broader population lacking distance and face-to-face learning resources. According to Smedley (2010), online education allows students to have a learning experience that is available anytime and anywhere they are currently located. A wide range of learning opportunities allows students to choose an education that best fits their learning style. Smedley, 2010 reports that it allows for a flexible pedagogy that gives students multiple avenues for support.
Deming, Goldin, Katz, and Yuchtman (2015) conducted a study to see if online education could assist in offsetting the cost for institutions. The researchers found that universities with a higher enrollment of online students could charge less per class than those institutions with lower online enrollment. Christensen and Eyring (2011) found similar results. The researchers reported that traditional universities have followed set standards and have been increasing in size. However, these universities have had to raise tuition to keep up with this increase. The researchers noted that because of this rise, online institutions can cater to a more significant number of students that traditional universities have become too costly for them to attend. Besides cost, Christensen and Eyring (2011) also pointed out that these students benefited from online education because they enjoyed the convenience of setting their schedule, living at home, and not having to quit their current job.
Drawbacks of Online Education
Although online education has several benefits, it comes with tradeoffs. Research has noted that technology is a major setback to online education (Hamdan, 2014; Keengwe & Kidd, 2010; Nkonge & Gueldenzoph, 2006). For example, lacking internet connection and low-quality computer hardware and programs can positively affect the online learning experience. However, more than just the technology can be the issue. Nkonge & Gueldenzoph (2006) indicated that teachers’ lack of technical experience, students’ procrastination or study habits, and poor new student online orientation contribute to unsuccessful online education programs.
Researchers have pointed out that a major setback to online education is the need for an immediate teacher presence (Talebian et al., 2014). Talebian et al. (2014) found that online education lacks lively communication between students and teachers without the traditional face-to-face learning model. The researchers also noted that the absence of a teacher comes with limited feedback for both teachers and students. Even though students and teachers can effectively communicate through online technology, it can be difficult, at times, to accurately assess the type of feedback being received. However, Talebian et al. (2014) noted that although online education has a few disadvantages, these issues are becoming less frequent with the rapid increase in technological advances.
Deming, Goldin, Katz, and Yuchtman (2015) conducted a study to see if online education could assist in decreasing the cost of tuition. They looked at both public and private four-year and two-year institutions. However, what is interesting about this study is that the researchers conducted a resume audit experiment where they created similar fictitious job applicants, one from an online institution and one from a traditional institution. The results showed that employers were less likely to contact online for-profit institutions than applicants from non-selective public institutions (Deming et al., 2015).
Online Education and Mental Health
It comes as no surprise that mental illness can negatively affect academic success for college students. Research shows that students who have a significant mental illness are less likely to complete their degree (Barr, 2014; Keys et al., 2012; Kessler et al., 1995). In 2017, The American College Health Assessment found that within the last 12 months, factors that students experienced that affected academic performance were anxiety (21%), depression (16.8%), alcohol or drug use (4.6%), and stress (31%). However, the survey did not distinguish between online and on-campus students. Often, student support assistance can be overlooked for distance learning students, and there is a lack of empirical data for guidelines on an effective delivery system for these services (LaPadula, 2003).
Mark Nichols (2010) conducted a mixed-method study to see if distance learning students' perceptions of support services were correlated with retention. In the study, the researcher produced two separate surveys, one in 2008 (n=51) with 28 total respondents and a follow-up survey in 2009 (n=44) with 23 total respondents of first-year and potentially at-risk students. After the surveys, respondents were asked to complete a follow-up interview. Nichols (2010) found that online students found it impossible to continue their education when their health, family, or employment situations changed. The researcher also found that students are less likely to attribute their dropout behavior to the lack of student support services. However, when there are adequate support services, they are more likely to attribute their success to personal motivating factors (2010).
Amy Raphael (2006) conducted a study to determine online students’ perceived needs for student services and if they were being met. The investigator surveyed 272 online students with an inventory consisting of 49 items. The study showed that students' most perceived needs were academic advising, while their least perceived needs were personal counseling. Raphael (2006) also found that students reported that their universities did not meet those perceived needs. It is important to note that the researcher believes students’ lack of perceived need for online counseling may have to do with students’ full-time employment. Many organizations have employee assistance programs (EAP) that offer personal counseling to their employees (Raphael, 2006).
Similarly to Raphael, Maria LaPadula (2003) found that online students were generally satisfied with the services they received but wished their universities offered more specialized services like other institutions. The researcher surveyed 92 online students and found that while being satisfied with services offered by the library and admissions, they expressed interest in social services, academic advising, technical issues, and mental health counseling. Those students went on to report wanting to have services such as time management, access to an online psychologist, and hotline numbers for mental disorders (LaPadula, 2003).
Method
This study used a Pearson R correlation to assess if graduate students in online counseling programs' anxiety levels are associated with academic performance. This design allowed us to ascertain if there was a relationship between anxiety levels and academic performance. The sponsoring institution’s Institutional Review Board provided permission to conduct this study. The study looked at graduate students currently enrolled in the Masters of Clinical Counseling program at Bellevue University. The researchers used the General Health Questionnaire (GHQ-28) to measure anxiety levels and collected participants' GPAs to evaluate academic performance.
Participants
Participants were recruited from Bellevue University, a private Midwestern university, in the winter of 2018. The inclusion criteria required participants to be graduate students enrolled in the Clinical Counseling program and aged 18 or older. The study's sample included a diverse range of participants categorized as follows: Non-Hispanic White or Euro-American (0), Black, Afro-Caribbean, or African American (1), South Asian or Indian American (2), East Asian or Asian American (3), Native American or Alaskan Native (4), Latino or Hispanic American (5), Middle Eastern or Arab American (6), and other (7). Gender was recorded as Male (0) or Female (1). Additional demographic details, including age and years in the program, were collected through self-report items on the demographic sheet.
Measures
Demographics. A demographic data sheet was used along with the survey instrument to ascertain the participants’ age, race/ethnicity, gender, and year in the program. The participants were classified into Non-Hispanic White or Euro-American (0), Black, Afro-Caribbean, or African American (1), South Asian or Indian American (2), East Asian or Asian American (3), Native American or Alaskan Native (4), Latino or Hispanic American (5), Middle Eastern or Arab American (6), other (7), Male (0), Female (1). The classification system used in this study came from a modified version of the current U.S. Census. The participant's ages and years in the program were also collected through self-report items on the demographic sheet.
Mental Health. The Mental Health Inventory from the Medical Outcomes Study measured participants' mental health. This inventory looks at an individual Cognitive Functioning, Psychological Distress, Depression, Anxiety, Positive Affect, and feelings of Belonging. General Health Questionnaire (GHQ-28), which is classified into four areas (depression, anxiety, physical symptoms, and disorder social performance) on a Likert scale from 0-3. The participants’ mental health score is the total score added to each item. There are a total of 28 items that are divided into four areas with seven questions each. The reported reliability coefficient was 0.96, while the reported subscales were depression (0.94), anxiety (0.90), physical symptoms (.89), and disorder in social performance (0.78) (Bahmani, 2006).
Academic Performance. Participants were asked to disclose their overall academic GPA on a 4.0 scale. Respondents were asked to remain anonymous to increase their truthfulness and sense of security.
Procedures
An independent T-test and Pearson correlation coefficient were used to analyze the data. Participants were emailed a link to an online survey, which all students enrolled in the master’s clinical counseling program at Bellevue University had the opportunity to complete. Only fully completed surveys were included in the analysis. Statistical analysis was conducted using SPSS-25 to examine the relationship between anxiety levels and academic performance.
Results
A Pearson Correlation was computed to examine the relationship between anxiety and Grade Point Average (GPA). Scale scores for the GHQ-28 were computed by recoding the data from a 1-6 range to a 0-100 range. The mean anxiety score was 25.2 (SD=5.7), and the mean GPA was 3.66 (SD=0.32). The correlation between anxiety and GPA was positive but weak and not statistically significant (r(121)=.024, p=.79). This indicates that the students’ self-reported anxiety levels were not significantly associated with their GPAs.
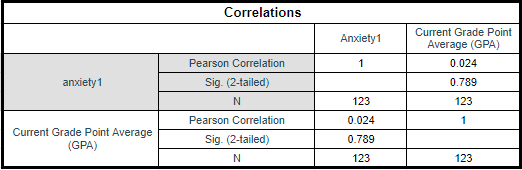
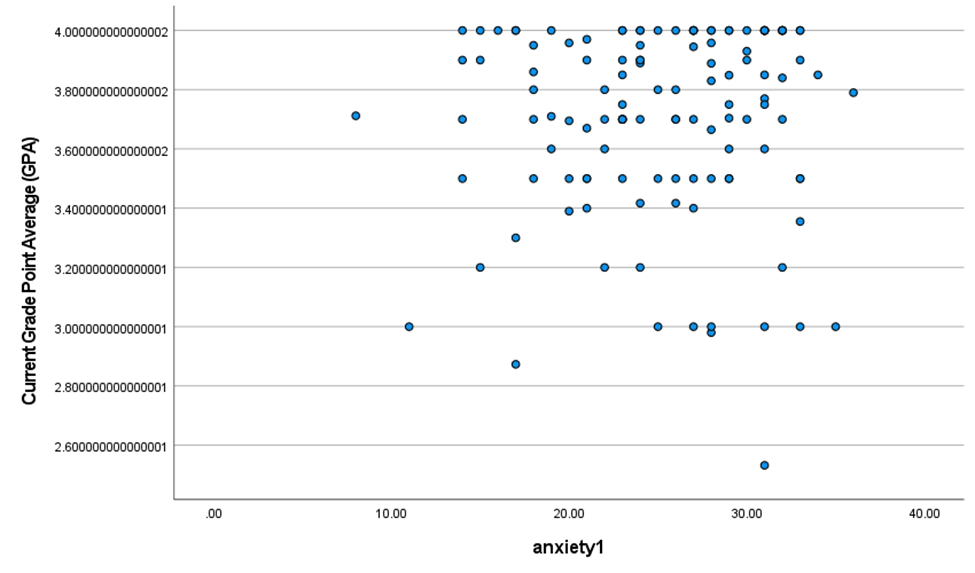
Discussion
The purpose of the study was to document the relationship between online counseling students’ perceived anxiety levels and their overall GPAs. Previous literature has shown that mental health can negatively affect academic success for college students (Barr, 2014; Keys et al., 2012; Kessler et al., 1995). This study investigated students’ academic success based solely on their GPAs. We cannot determine whether these students continued to succeed in college; however, the findings were somewhat inconsistent with previous research in that their mental health was not a strong indicator of academic success. Almost half of the participants reported feeling rattled, upset or flustered within the past month when the study took place, which goes back to the original topic of higher levels of anxiety among college students.
Looking at the correlation between anxiety and students’ GPA, the findings failed to reject the null hypothesis. In this study, the occurrence of Anxiety, Depression, and psychological distress were found to be low levels in more than half of the students surveyed.
Results
The purpose of this study was to document the relationship between online counseling students’ perceived anxiety levels and their overall GPAs. Previous literature has shown that mental health can negatively affect academic success for college students (Barr, 2014; Keys et al., 2012; Kessler et al., 1995). However, our findings did not reveal a statistically significant correlation between anxiety levels and academic performance, as measured by GPA (r(121) = .024, p = .79). This lack of significant results suggests that anxiety may not be as influential on academic outcomes in this specific population as previously thought. It is possible that other variables, such as time management skills, support systems, or coping mechanisms, play a more critical role in academic success for online graduate students. Future research should explore these additional factors to gain a more comprehensive understanding of what influences academic performance in online educational settings. Our study highlights the complexity of factors affecting student performance and underscores the need for a multifaceted approach to supporting online learners.
Further Research
Future research should explore additional variables that may influence the relationship between anxiety levels and academic performance in online education settings. Specifically, examining factors such as time management skills, support systems' effectiveness, and coping mechanisms' role could provide a more comprehensive understanding of student success in digital learning environments. Longitudinal studies tracking students over time would help identify changes in anxiety levels and academic outcomes, offering insights into the long-term impact of online education. Additionally, investigating the efficacy of various mental health support interventions tailored for online learners could inform best practices for educational institutions. Comparative studies between traditional and online education formats regarding mental health and academic performance further elucidate digital pedagogy's unique challenges and advantages.
References
American College Health Association. American College Health Association-National College Health Assessment II: Reference Group Executive Summary Fall 2017. Hanover, MD: American College Health Association; 2018.
Arkorful, V., & Abaidoo, N. (2015). The role of e-learning, advantages and disadvantages of its adoption in higher education. International Journal of Instructional Technology and Distance Learning, 12(1), 29-42.
Bahmani, B., & Askari, A. (2006). National normative data and psychometric properties of mental health questionnaire for medical students of Iran. In Proceeding of the National Congress of student’s mental health.
Baron, K., Fritz, J., & Mozie-Ross, Y. (2023). Inclusive excellence online: Pandemic lessons learned supporting traditionally underserved students. Advances in Online Education: A Peer-Reviewed Journal, 2(1), 60-70.
Barr, B. (2014). Identifying and addressing the mental health needs of online students in higher education. Online Journal of Distance Learning Administration, 17(2).
Bransford, J. D., Sherwood, R. D., Hasselbring, T. S., Kinzer, C. K., & Williams, S. M. (1990). Anchored instruction: Why we need it and how technology can help. In D. Nix & R. J. Spiro (Eds.), Cognition, education, and multimedia: Exploring ideas in high technology (pp. 115-141). Hillsdale, NJ, US: Lawrence Erlbaum Associates, Inc
Christensen, C. M., & Eyring, H. J. (2011). The innovative university: Changing the DNA of higher education from the inside out. John Wiley & Sons
Deming, D. J., Goldin, C., Katz, L. F., & Yuchtman, N. (2015). Can online learning bend the higher education cost curve?. American Economic Review, 105(5), 496-501.
Di Giovanni, L., & Cronin-Gilmore, J. (2024). The transition from traditional to online education for the adult learner. Advances in Online Education: A Peer-Reviewed Journal, 2(4), 294–302.
Fedynich, L. V. (2013). Teaching beyond the classroom walls: The pros and cons of cyber learning. Journal of Instructional Pedagogies, 13.
Giokaris, I. (2024). Evaluating distance education for students with special needs in Greece and Cyprus: Insights from an online learning survey. Advances in Online Education: A Peer-Reviewed Journal, 2(4), 351-371.
Hamdan, A. K. (2014). The reciprocal and correlative relationship between learning culture and online education: A case from Saudi Arabia. The International Review of Research in Open and Distributed Learning, 15(1).
Harvey‐Jones, V., Koekkoek, S., Adair, G., & Craddock, C. (2022). PB1780: An innovative interactive case-based online educational tool substantially increases knowledge and competence of clinicians managing high-risk AML. HemaSphere, 6(Suppl), 1660–1661. https://doi.org/10.1097/01.HS9.0000849972.53767.90
Hatahet, T., Raouf Mohamed, A. A., Malekigorji, M., Clerkin, C., & Sandrey, L. (2024). Personal and engagement factors in remote learning contributing to high student achievement in a transnational education context: A quantitative study. Advances in Online Education: A Peer-Reviewed Journal, 2(4), 330–350.
Hu, Y. L., Ching, G. S., & Chao, P. C. (2012). Taiwan student engagement model: Conceptual framework and overview of psychometric properties. International Journal of Research Studies in Education, 1(1), 69-90.
Keyes, C. L., Eisenberg, D., Perry, G. S., Dube, S. R., Kroenke, K., & Dhingra, S. S. (2012). The relationship of level of positive mental health with current mental disorders in predicting suicidal behavior and academic impairment in college students. Journal of American College Health, 60(2), 126-133.
LaPadula, M. (2003). A comprehensive look at online student support services for distance learners. The American Journal of Distance Education, 17(2), 119-128.
LaPointe, L. & Reisetter, M. (2008). Belonging Online: Students' Perceptions of the Value and Efficacy of an Online Learning Community. International Journal on E-Learning, 7(4), 641-665. Waynesville, NC USA: Association for the Advancement of Computing in Education (AACE). Retrieved September 16, 2024 from https://www.learntechlib.org/primary/p/24419/.
Lloyd, C., Herb, A., & Kilmister, M. (2023). Connecting the pages: Aligning online course development with academic professional development for student success in online learning. Advances in Online Education: A Peer-Reviewed Journal, 1(3), 296-307.
Nichols, M. (2010). Student perceptions of support services and the influence of targeted interventions on retention in distance education. Distance Education, 31, 93-113.
http://dx.doi.org/10.1080/01587911003725048
Nkonge, B., & Geuldenzolph, L. (2006). Best practices in online education: Implications for policy and practice. Business Education Digest, 15, 42-53.
Pelz, B. (2010). (My) three principles of effective online pedagogy. Journal of Asynchronous Learning Networks, 14(1), 103-116.
Pike, G. R. (1999). The effects of residential learning communities and traditional residential living arrangements on educational gains during the first year of college. Journal of college student development, 40(3), 269.
Raphael, A. (2006). A needs assessment: A study of perceived need for student services by distance learners. Online Journal of Distance Learning A
Rezaei, A. R. (2024). Applications of immersive technologies in education: A systematic literature review. Advances in Online Education: A Peer-Reviewed Journal, 2(3), 232-251.
Sanda, M.-A. (2024). Influence of instructors’ adaptability and management of virtual classroom environments on the effectiveness of tertiary students’ virtual knowledge acquisition. Advances in Online Education: A Peer-Reviewed Journal, 2(3), 213-225.
Shea, P. (2006). A study of students’ sense of learning community in online environments. Journal of Asynchronous Learning Networks, 10(1), 35-44.
Smedley, J. (2010). Modelling the impact of knowledge management using technology. OR insight, 23(4), 233-250.
Stassen, M. L. (2003). Student outcomes: The impact of varying living-learning community models. Research in higher education, 44(5), 581-613.
Stephens, W. (2023). Augmented and virtual realities for distance teaching and learning. Advances in Online Education: A Peer-Reviewed Journal, 1(4), 357-362.
Talebian, S., Mohammadi, H. M., & Rezvanfar, A. (2014). Information and communication technology (ICT) in higher education: advantages, disadvantages, conveniences and limitations of applying e-learning to agricultural students in Iran. Procedia-Social and Behavioral Sciences, 152, 300-305.
Tao, Y. H., Yeh, C. R., & Sun, S. I. (2006). Improving training needs assessment processes via the Internet: system design and qualitative study. Internet Research, 16 (4), 427–49.
Zhao, C. M., & Kuh, G. D. (2004). Adding value: Learning communities and student engagement. Research in higher education, 45(2), 115-138.